Astrocyte
is a code-free AI training tool to quickly deploy AI models for
machine vision tasks. It allows users to use their own images
of products, samples, and defects to train neural networks to
perform applications like anomaly detection, classification, object
detection, segmentation, and noise reduction.
With its highly flexible graphical user interface (GUI), Astrocyte
allows visualizing and interpreting models for performance and
accuracy as well as exporting these models to files that are ready
for runtime in Teledyne Dalsa's Sapera and Sherlock vision software
platforms.
In this version of Astrocyte (1.30),
the following key features have been introduced:
Tiling on Large Images
Tiling is the process by which images can be analysed to obtain
detailed insights into small details within large images. Tiling
allows applying training and inference on native resolution without
having to downscale (as with other AI software) which can cause
loss of detail or memory overload. In Astrocyte 1.30 the tiling
workflow consists of decomposing an input image into sub-images
(tiles), iteratively processing the tiles at native resolution,
and recombining the tiles at the output. The tile size is selected
either automatically or manually.
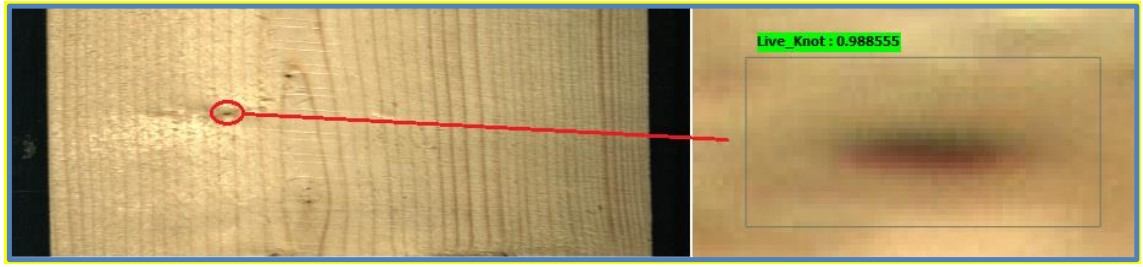
In the example
above, tiling is used to identify knots on wooden planks. The
input image has a resolution of 2800 x 1024 and contains knots
with sizes as low as 10 pixels.
Increased
Performance on Anomaly Detection
Astrocyte 1.30 introduces a new Anomaly Detection algorithm providing
better performance on low to medium resolutions compared to the
previous version. Use Anomaly Detection directly on images ranging
from 256 x 256 to 1024 x 1024. For resolutions above 1024 x 1024
you can combine Anomaly Detection with tiling or decimation. This
new algorithm replaces the old pixel-level algorithm
and is the new default. The old algorithm is preserved for legacy
purposes.
Anomaly Detection does not need annotations at training, making
it easier to train than object detection and segmentation. Anomaly
Detection can locate any defect never seen at training given the
training is performed on good samples only.
Below is an example of Anomaly Detection usage. The left-hand
third shows a normal image with no defects. In the middle and
the right images, are different types of defects (highlighted
with pseudo-colour). The challenge in this example is to differentiate
the defects from the normal lettering.
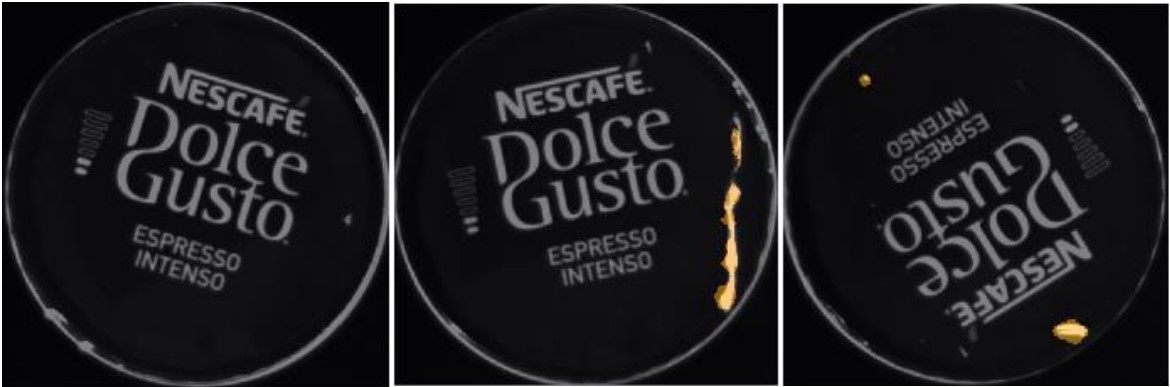
YOLOX Object Detection
Astrocyte
1.30 offers YOLOX, an additional object detection algorithm. It
is more compact and offers improved performance capabilities than
the existing SSD (Single Shot Detector) algorithm. Because of
its compactness, YOLOX is widely used in embedded devices.
Processing of Non-Square Images
Astrocyte now uses a flexible mechanism for preparing the input
image before training and inference. The image sent to the neural
network can have different width and height. This ensures preserving
the original aspect ratio (and, therefore, avoid distorting the
image with stretching or padding). At training time, you have
the option of using either the original, decimated, or tiled input
image.
Other General Improvements
Now supports frame-grabbers allowing Astrocyte
to acquire live images from any Teledyne Dalsa device
New diagnostic report to help troubleshooting training
issues at customer sites
Dynamic discovery of cameras (automatic detection
of plug/unplug GigE/USB3 cameras)
Saving datasets as TAR format to allow altering
and merging previously created datasets
Improvement of training progress display (now displays
both epoch numbers and steps)
Improvement of brush tool for segmentation annotations
Click
here to find out more about Astrocyte: